
AI is the Future. ChatGPT is the assistant.
The hype cycle of ChatGPT has significantly raised the level of awareness about AI technologies. ChatGPT has been hailed as one of the most significant breakthroughs in AI in recent years. It is a large language model (LLM) that has the ability to generate text that is virtually indistinguishable from that produced by a human. This has led to a great deal of excitement and anticipation around AI technologies.
The hype around LLMs reached its crescendo when Elon Musk and Apple co-founder Steve Wozniak called for a six-month pause to consider the risks. This heightened level of awareness has raised the level of importance and urgency for businesses to have a thoughtful plan for how they will (and won’t) be using AI across all functions.
Now more than ever, AI is within arms reach of being useful for every person, every business, and every function of the business. Large language models like ChatGPT, Google's PaLM, Bard, and LLaMa (to name a few) have made that happen due to their general usefulness across many tasks like search, question and answer, coding, and generating content like emails or blogs. It’s likely that anyone reading this has played with some LLMs. By now you get it. Businesses, however, do not. It is far from obvious how this translates to the business world.
While we have seen the power of AI to increase revenue, increase operational efficiency and increase the overall value of businesses, many businesses find it difficult to measure returns from AI spending. Even today, according to various studies, the percentage of AI projects that fail to deliver their intended outcomes is as high as 50% to 85%. This is due to a variety of factors, including the lack of a clear understanding of the business problem or use case, overlooking the human need and interaction with AI, inadequate data quality or quantity, and a lack of talent or expertise. While the cost of AI projects can be small, significant investment in time, budget, and resources is required to see the full value of AI transformation. Executives and team members must be committed to investing in AI and must be prepared to accept that not all AI projects will succeed. To succeed in AI, you must be prepared and accepting of failure, learning, iteration and continuous improvement.
To see success in AI, we need a better paradigm. Adding LLMs and in AI in general should be seen through the lens of adding a large team of analysts or personal assistants. Thinking this way will accelerate the adoption and steer your strategy on where, when, and how to deploy it successfully.
AI, like people, learns. AI is not static in capability, but in fact gets more performant over time as it sees more data. We train models in a similar way we train. Through experience and being corrected when we make mistakes, models learn in a similar way. For example, the same code that may be created to identify cats in an image can be retrained to identify cancer in a mammogram. AI also makes mistakes. As do we. AI takes actions based on confidence. The higher the confidence is a measure of the level of training the model has. The level of training depends on the data available to train the model. Sound familiar?
Because we are human and fallible, we are on a life-long journey of growth and development. To help facilitate this in the workplace, we have managers. Managers train their employees, correct mistakes, and use input from their teams to make strategic decisions. AI and LLM like technology to be successful in the workplace needs managers too. We call this a human-in-the-loop.
To be successful in AI and take advantage of ripe opportunities to leverage models like LLMs and ChatGPT you need to have the same mindset of hiring a new employee. But this employee is closer to an analyst or assistant than your next CEO. Therefore businesses must change their mindset. AI can be transformational even when it makes mistakes, lacks confidence, or can only take on very minute tasks.
Like an assistant, AI should be relegated to simple tasks; data entry, research, basic data analysis, and or recommendations. With ChatGPT, it can be trusted to help write reports or summarize information. Like an assistant, it cannot fully automate your job, make final strategic decisions, or even devise a strategy. Like an assistant, it needs management (us) to manage it, train it, correct mistakes, and take the input to make final decisions. It can be trusted to give you time back by taking on mundane routine tasks to accelerate your productivity. And it can do it today.
Let me give you an example. ChatGPT wrote 50% of this blog. Here is how I did it:
- I started with an outline:
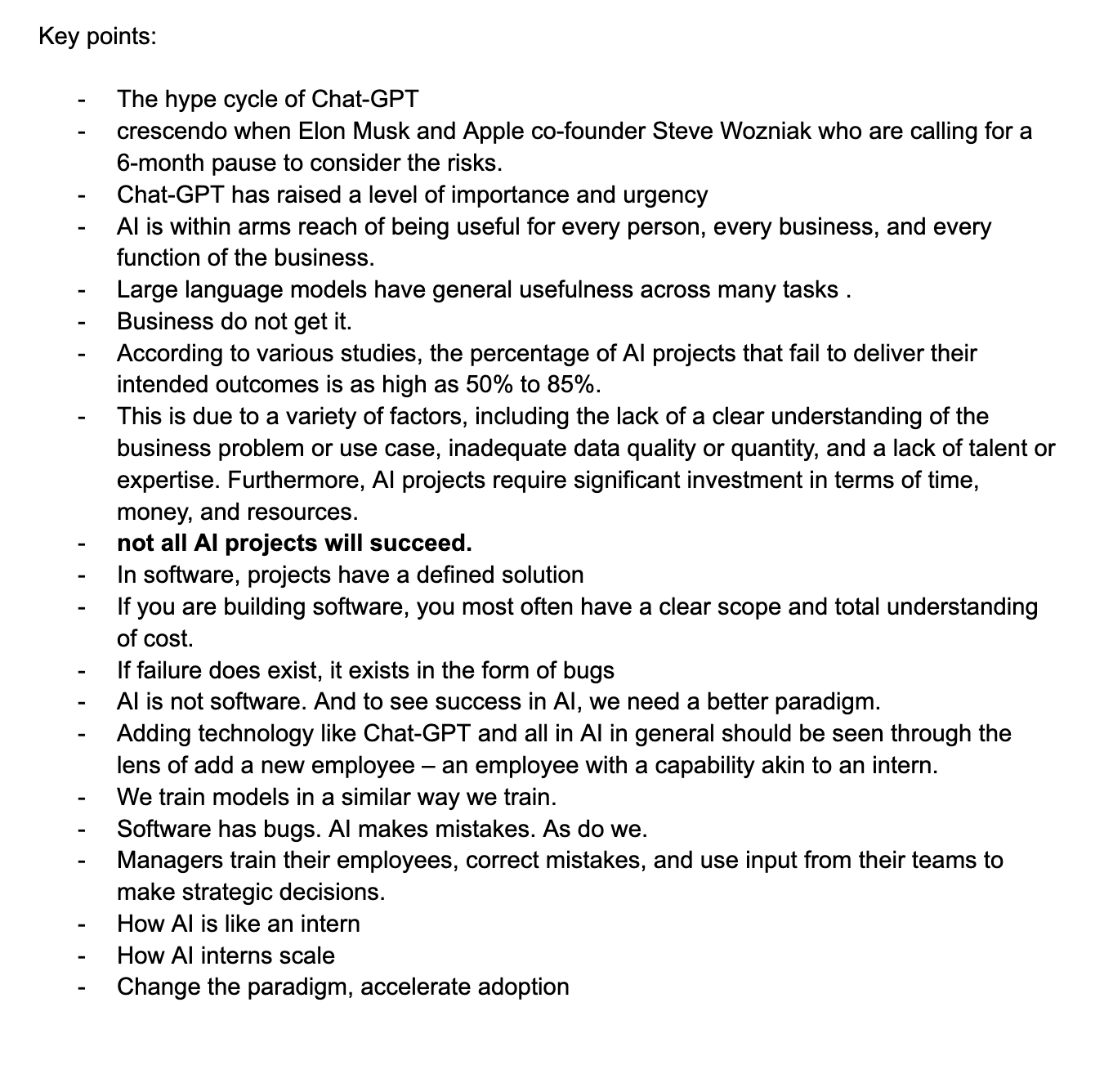
- I asked ChatGPT to write me a blog that hits on these key points:

- I read it and realized I need a longer blog.

- It made a mistake. I asked why. It gave me some advice.
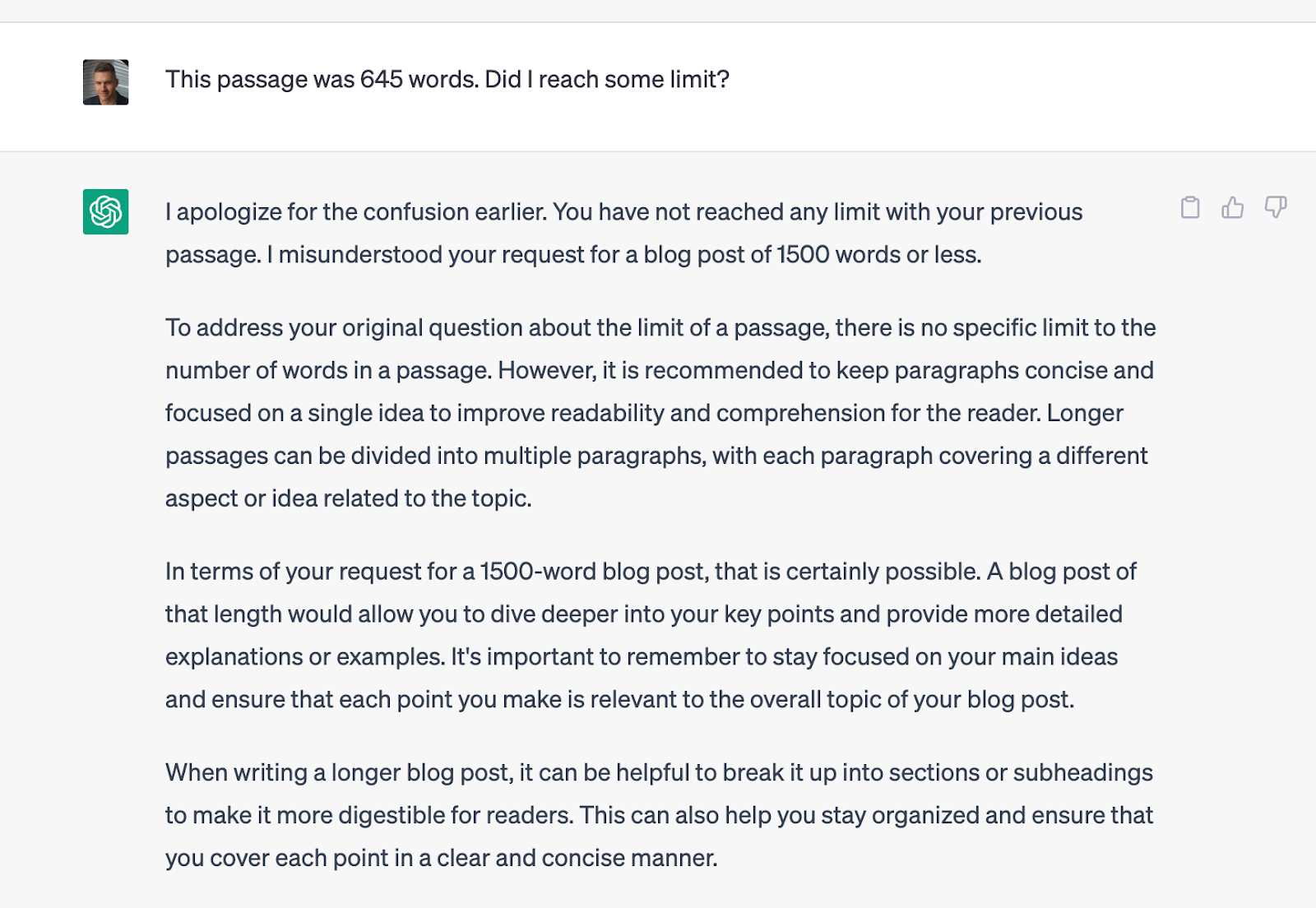
- I asked again. It really missed the mark.

- I stopped it. I tried again.

- We have an acceptable draft! I asked for some headers (I took it’s advice).
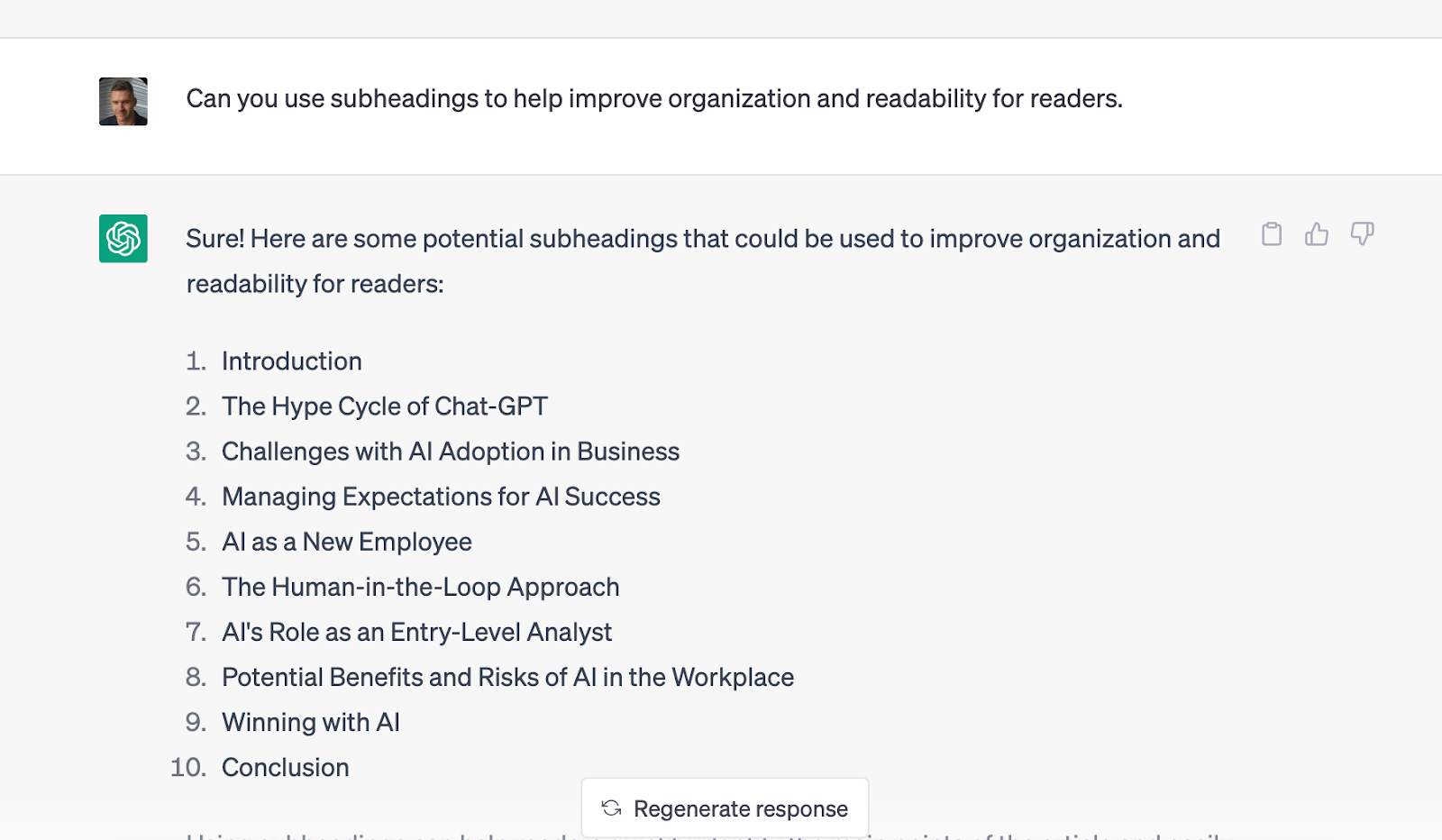
- I made copy edits and finalized.
- Done. (Total time ~1 hour)
ChatGPT is not perfect. Like us, it causes issues. Unfortunately, like us it can lie. It can be offensive. It may cause issues with the greater team, lack or lose trust, and be ignored because it is intimidating. These are management issues, HR issues, and culture issues. However we can manage these issues if we are prepared to manage them. Given all the issues, we still need assistants. And if we apply the same thinking to our AI, we need them too. In fact, businesses in your industry are competing and winning on their AI, whether you know it or not.
The upside of AI is the unlimited ability to learn, remember, and scale. “Your-GPT” is not a single employee, it has the ability of thousands of employees who can bend time and give you output instantly that would otherwise take a month, or a year. If we start thinking about AI as hiring an employee, we will start to see the role of AI in a better light. It forces businesses to consider what types of roles are best suited for a learning algorithm that can make mistakes. And how it might help and enable its users to do more with less. Most importantly, it will help us consider who fits into the larger organization, how to we train it, address issues as they arise, and keep the culture intact.