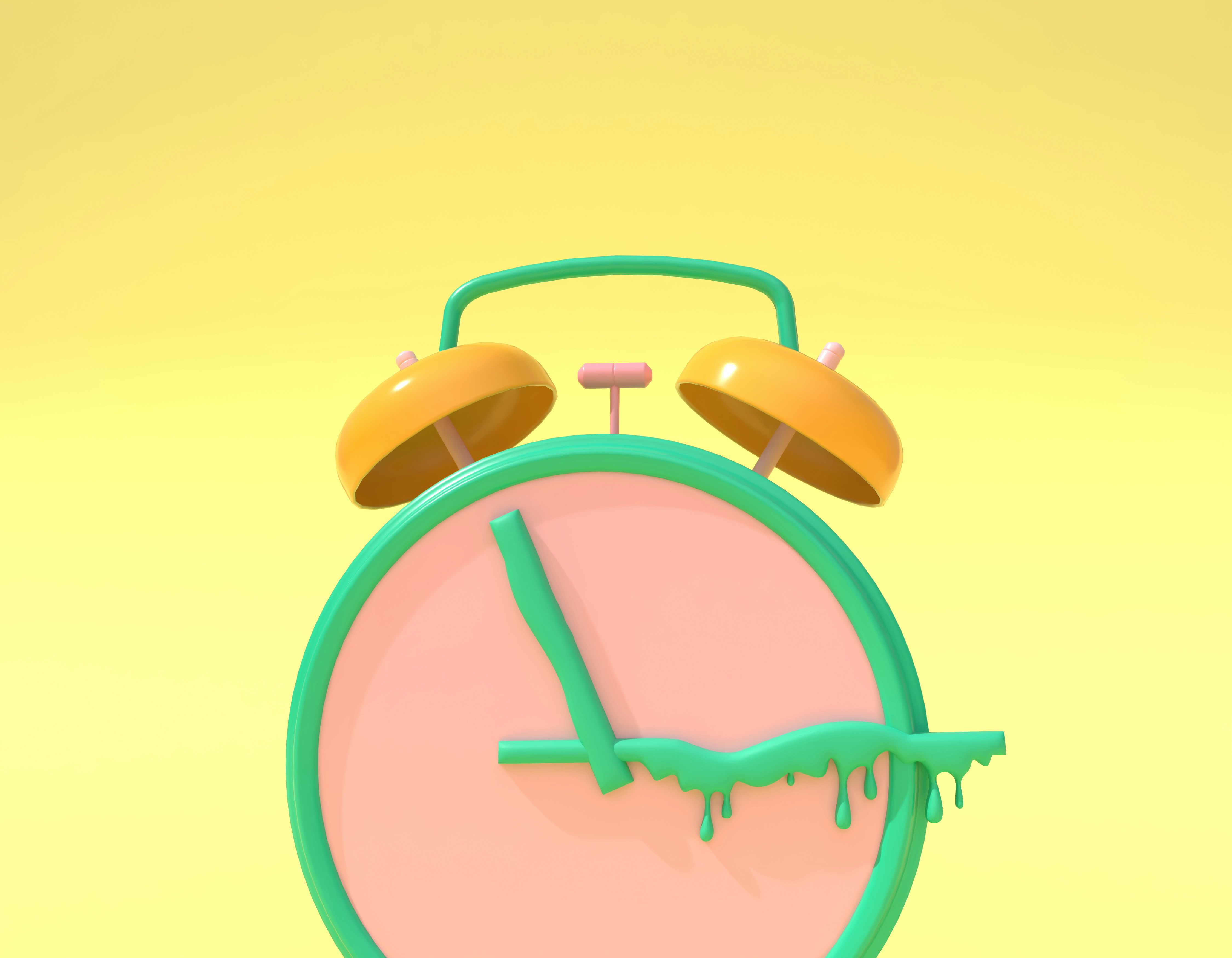
What Does “Productivity” Mean in an AI-Enabled World?
Insights from our third CO.LAB session
Recently, we hosted the third session of CO.LAB, KUNGFU.AI's futures-oriented co-design series where we explore the evolving relationship between humans and AI. Held during our weekly Lab Day—a space for staying ahead of rapid advancements in our field—this session built on our previous discussions about reclaiming attention and authenticity, and tackled a fundamental question:
In a world where AI can outperform humans in tasks demanding speed, scale, and efficiency, is productivity still a useful metric—particularly for knowledge industries?
Rather than viewing productivity through the narrow lens of output maximization or debating AI's impact in deterministic terms, CO.LAB reframes this conversation as a design challenge. What follows is a recap of our discussion.
The Productivity Paradox
We began our session by examining the historical context of productivity. Humans have dramatically increased mechanical productivity over millennia—from muscle power to horsepower to modern engines with exponentially greater capabilities. For instance, while an average human can sustain about 80 watts of power output, a single horsepower equals ~745 watts—nearly 10x that of a human. Modern industrial engines can produce thousands of horsepower, representing a mechanical productivity gain of several thousand-fold over human muscle power alone. The transition from draft animals to mechanized farming in the early 20th century provides a clear example, where a single tractor could do the work of dozens of horses (and their human handlers).
Yet modern productivity gains haven't kept pace, increasing only about sixfold in the last 25 years compared to the hundredfold gains in mechanical domains.
This gap reveals a fundamental challenge: we've applied industrial-era productivity frameworks to fundamentally different types of work. As one participant noted, "In a factory setting, productivity means hours or labor savings. The conveyor belt made automobile assembly more efficient and therefore productive. But in the modern economy, productivity is far more aligned with non-time-based productivity like the creation of entirely new categories of products and services."
In other words, measuring the quantity of output makes sense for standardized production, but as an approach it’s insufficient when applied to knowledge work where quality, creativity, and impact matter most.
When Optimization Becomes Dehumanizing
Our discussion quickly surfaced concerns about the modern-day obsession with productivity optimization, or "productivity hacking." From LinkedIn influencers sharing their 4 AM routines to apps that track every metric of our existence, we're increasingly encouraged to optimize every moment of our lives. Setting aside whether or not this is healthy (in the extreme, it’s not), it’s worth asking: is this even helpful?
One participant reflected on the philosopher Josef Pieper's notion that "leisure, it must be remembered, is not a Sunday afternoon idyll, but the preserve of freedom, of education and culture, and of that undiminished humanity which views the world as a whole." This perspective suggests that our fixation on productivity might actually undermine one of our most distinctly human capabilities: our ability to see connections across domains and (at least partially) comprehend complex systems holistically.
In his influential work Leisure: The Basis of Culture, written in post-World War II Germany, Pieper warned that a society focused exclusively on work risks losing something fundamental to human flourishing. He defined leisure not as idleness but as "the disposition of receptive understanding, of contemplative beholding, and immersion in the real"—precisely the mental state from which innovation often emerges.
"What humans value changes over time," observed another participant. "And part of the way that it changes is based on what's perceived as high-effort versus low-effort." Throughout history, what we consider valuable work has shifted as technology has automated different types of labor. The question now is whether our definition of productivity will evolve to value the uniquely human contributions that AI cannot (yet) replicate, including those nurtured and sustained by behaviors antithetical to productivity hacking.
The Cross-Disciplinary Advantage
A particularly compelling insight emerged around the relationship between specialization and human value in an AI-enhanced world. "There's this generalist-specialist spectrum," one participant noted. "The knowledge workforce often pressures people to specialize... but a lot of the biggest innovations come from cross-discipline thinking."
To this end, research has consistently shown that breakthrough innovation often happens at the intersection of disciplines. According to studies in innovation management, teams with diverse expertise are 1.5 times more likely to outperform their competitors by developing solutions that incorporate multiple perspectives. This "cross-pollination" of ideas can lead to unexpected connections that drive transformative advances impossible within siloed disciplines.
While AI systems excel at specialized tasks within defined domains, humans uniquely contribute through connecting disparate fields and ideas. This capacity for cross-disciplinary insight—seeing patterns across seemingly unrelated domains—may represent one of our most valuable cognitive abilities in an AI-driven future. Even as large language models become increasingly adept at drawing connections between disparate subjects, human discernment and taste remain essential for evaluating which connections are truly meaningful and worth pursuing.
As one participant observed, "Specialists kind of do the best within the existing system, while generalists change the system." In a world where AI increasingly handles specialized tasks, human productivity might be better measured by our ability to reimagine systems rather than optimize within them.
Reclaiming Educational Models
Our discussion also revealed how deeply our productivity mindsets are shaped by educational systems that prioritize assignment completion over problem identification and solution. "We're trained all of our formative years that if we're given an assignment, we need to complete that assignment," one participant observed. "So there's a real drive when we enter the workforce to, 'Oh, I was given a task, therefore it must be done.'"
This conditioning leaves many knowledge workers ill-equipped for the most valuable contribution in an AI-enhanced world: defining problems worth solving rather than efficiently executing predefined tasks. Several participants envisioned a different educational model—one centered on "identifying and solving real problems in your community" rather than "ingesting tasks given to you and spitting back out answers."
Such education would prepare people for a world where AI handles more routine execution, while humans focus on framing questions, identifying opportunities, and navigating ethical complexities.
What Becomes of ‘Bullshit Jobs’?
Our conversation took an interesting turn as participants reflected on how the modern economy often creates what anthropologist David Graeber called "bullshit jobs"—roles that even those performing them privately question the value of. One participant shared an anecdote about a friend who worked at a call center contacting people who had been searching for mortgage brokers.
"There was no real societal benefit," the participant reflected. "There was fractional return on investment benefit, but there was no societal benefit to that labor being spent." This observation highlights a troubling disconnect: while such jobs might register as "productive" in conventional (shareholder-maximizing financial) metrics, they fail to create meaningful value or contribute to human flourishing.
In his influential 2018 book Bullshit Jobs: A Theory, Graeber defined these roles as a form of employment that is “so completely pointless that even the person who has to perform it every day cannot convince themselves there’s a good reason for them to be doing it” (source). His research suggested that up to 40% of workers secretly believe their jobs don't need to exist (source). Graeber identified five types of meaningless jobs: flunkies (who make others feel important), goons (adversarial roles like telemarketers), duct tapers (who fix problems that shouldn't exist), box tickers (who create the appearance of useful activity), and taskmasters (who create or manage unnecessary work) (source).
As AI automates more routine tasks, this tension will likely intensify. The challenge isn't just technological but philosophical: how do we define productive work in ways that align with genuine human needs rather than simply perpetuating existing economic structures?
Time vs. Intention: A New Framework
Perhaps the most provocative reframing of productivity came toward the end of our session. One participant shared how many of history's great thinkers structured their days not around maximizing output, but around alignment with intention. "I think a better definition of productivity," this participant suggested, "is how closely aligned your lived actions are with what you set out to do at the beginning of the day, week, month, etc."
This shifts productivity from a pure output metric to one that centers human agency and intentionality. "So many of our days are constructed passively," the participant continued. "We look at our phones, we're fed content, we react to messages on Slack... if you actually reflect on what you did during the day versus what you set out to do, there's probably a great degree of divergence."
This insight suggests a fundamental reorientation: true productivity isn't about maximizing output, but aligning actions with intentions. Rather than measuring how much we produce, perhaps we should measure how deliberately we navigate our lives and work.
Designing for Human Flourishing
What would systems that enhance rather than diminish human flourishing look like? Our discussion yielded several design principles:
- Intentionality-Centered Metrics. Moving beyond output volume to measure how well we align our actions with our deeper intentions and values.
- Cognitive Diversity Support. Creating environments that value both specialized expertise and cross-disciplinary thinking, rather than forcing everyone into narrow specialization.
- Time for Synthesis. Designing workflows that incorporate deliberate space for reflection, connection-making, and system-level thinking.
- Balanced Efficiency. Recognizing when efficiency improvements should translate to reduced working hours rather than expanded scope, preventing the "race to the bottom" dynamic.
At KUNGFU.AI, we believe these approaches can't be implemented through technological determinism—they require intentional design choices that center human values and experiences.
Open Questions for the Future
As with any rich discussion, we left with as many questions as answers:
- How will our perception of "valuable work" evolve as AI assumes more specialized cognitive tasks?
- Can we develop new organizational metrics that better reflect meaningful human contribution in AI-enhanced environments?
- How might we redesign educational systems to prepare people for a world where cross-disciplinary thinking becomes more valuable than specialized execution?
- What cultural shifts are needed to allow efficiency gains to translate into more deliberate, intention-aligned use of time rather than just expanded workloads?
We'll continue exploring these questions in future CO.LAB sessions, focusing on how we might design AI systems that enhance human capabilities while preserving what makes us distinctly human.
If you're thinking about how to redefine productivity in your organization to better align with human flourishing in an AI-enhanced world, I'd love to collaborate. Let's build a future where technology serves human intention rather than narrowly defined efficiency metrics. You can reach out to me directly at ben.szuhaj@kungfu.ai.
Please note that the dialogue represented from the CO.LAB discussion in this piece has been edited for clarity and brevity. Additionally, I would like to explicitly acknowledge the use of AI in the creation of this piece—from helping to transcribe the session to providing support during my writing process.